All You Need To Know About Data Quality?
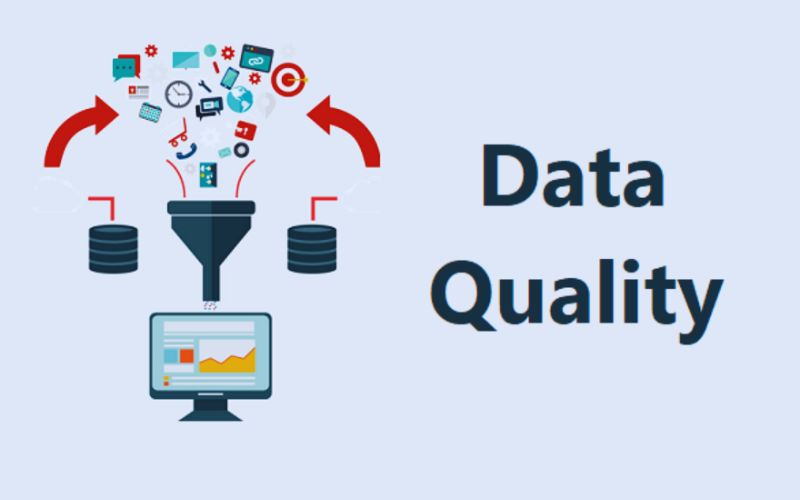
All You Need To Know About Data Quality?
Did you know that typical data quality issues affect over 90% of businesses? The most common are outdated contacts, incorrect dates, duplicates, and incomplete records. It will be pretty challenging to identify new customers and understand customer requirements and marketing development if the data is not clear and precise.
Table of Contents
Define Data Quality?
Data quality is essential to data governance that helps ensure your organization’s data is fit for purpose. Data quality refers to the practicality of a data set and ease of processing and evaluation for other purposes. Dimensions of data quality assurance such as accuracy, compliance, completeness, consistency, and completeness help ensure your data governance analyses consistently deliver reliable results.
A study found that human error is the primary reason for insufficient data today. Trying to improve poor-quality data takes a lot of time and effort. Other aspects that can lead to poor dq include miscommunication and wrong data management techniques.
How might poor data quality harm your business?
- Unreliable Analysis: Lower confidence levels in analysis and reporting make it quite challenging to manage results.
- Increased processing effort: Once the data is wrong, it will take ample time to complete a unit of work.
- Loss of brand value: A company’s brand value will quickly decline if frequent mistakes occur.
- Compliance Risk and Poor Governance: Compliance will no longer be optional and challenging to compete without.
How can data quality be assessed?
You will have no problem recognizing the data quality control. You will find it difficult to make precise assessments because the data quality is unclear. It can use many variables to achieve the ideal measurement technique and context for DQ.
Customer information must be accurate, complete, and accessible during a marketing campaign. Additionally, for a typical marketing campaign, customer data must be accurate, unique, and consistent at all levels of engagement. The DQ dimensions relate to the specific characteristics of the situation.
What aspects of data quality are there?
Accuracy: Companies need to represent actual events and situations in the data. Specialists should rely on confirmable sources to confirm the measure of correctness.
Consistency: Data consistency refers to data consistency as it comes from different sources and is go with across networks and applications. Character is vital because similar records should exist in other places without conflicts. It is important to note that consistent data can be erroneous.
Completeness: Data integrity assesses whether you can successfully provide the required values.
Uniqueness: Each information or event in a data set must be unique. There should be no duplicate records in the registry. Businesses can use data cleansing to help with a low uniqueness score.
Timeliness: Timely data is information that is voluntarily available when needed. This factor also requires keeping data current, up-to-date, and accessible at all times.
Validity: Companies must collect data within the parameters and regulations established by the company. Also, all data values must be in the correct range, and each registry value must conform to acceptable formats.
Also read: MSP Marketing Efforts
Also read: Keywords For Global SEO Campaigns